# 세줄 요약 #
- To develop and validate a deep convolutional neural network (CNN) method capable of (1) selecting a specific shoulder sagittal MR image (Y-view) and (2) automatically segmenting rotator cuff (RC) muscles on a Y-view.
- For model A, we manually selected shoulder sagittal T1 Y-view from 258 cases as ground truth to train a classification, For model B, we manually segmented subscapularis, supraspinatus, and infraspinatus/teres minor on 1048 sagittal T1 Y-views.
- A showed top-3 accuracy > 98% to select an appropriate Y-view and accurate RC muscle segmentations with mean Dice scores > 0.93.
# 상세 리뷰 #
1. introduction
- Rotator Cuff (RC) tendon tears are associated with varied degrees of muscle atrophy, manifested by decreased muscle bulk and fatty infiltration.
- MRI is the reference standard for imaging RC tendons for tears, severity of cuff abnormalities, and postoperative healing.
- We need accurate and automated segmentation strategies for estimation of RC muscle atrophy.
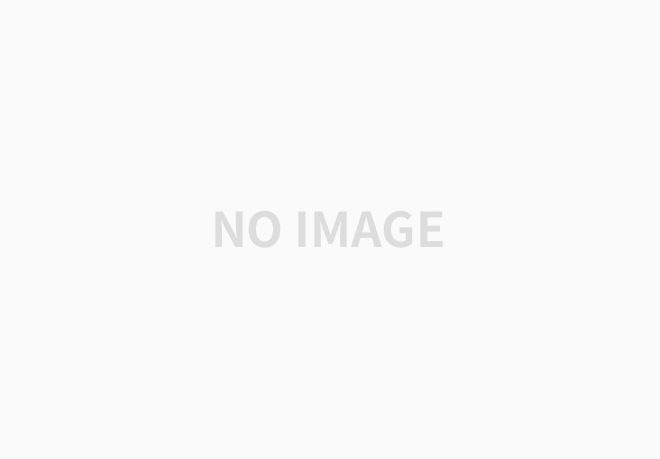
2. Method
- The purpose of our study was to develop deep convolutional neural networks (CNN) to identify a scapular Y-view.
- Our study was IRB-approved Dataset
- internal dataset
- Institution: General Electric, Waukesha, WI, USA; Hitachi Medical Corporation, Tokyo, Japan; Siemens Healthcare, Erlangen, Germany; Philips Healthcare, Amsterdam, Netherlands
- Duration: 2018.10 ~ 2020.01
- external dataset
- non-affiliated imaging facilities that were uploaded to our hospital's database.
- internal dataset
- We created two models.
- Model A. Y-view selection = classification CNN
- Inception V3 (16 batch, 100 epochs, droput 0.2, learning rate 0.001, optimizer RMSprop)
- train dataset: 258 cases, test dataset: 100 internal, 50 external cases.
- Model B. RC muscle segmentation = segmentation CNN
- modified U-Net (8 batch, 50 epochs, dropout 0.25, learning rate 0.0001, softmax)
- train dataset: 1048 images, test dataset: 105 internal, 50 external images.
- Model A. Y-view selection = classification CNN
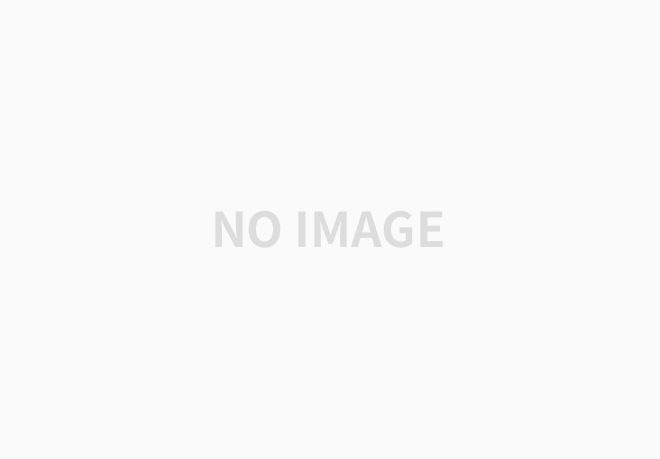
3. Result
- Model A (Y-view selection). top-3 accuracy 98%
- internal test cases: 98.7 ± 1.0%
- external test cases: 99.7 ± 1.0%
- Model B (RC muscle segmentation). mean Dice scores > 0.93
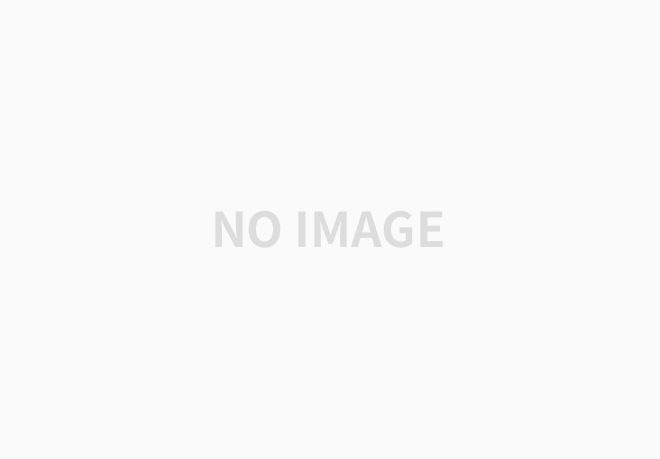
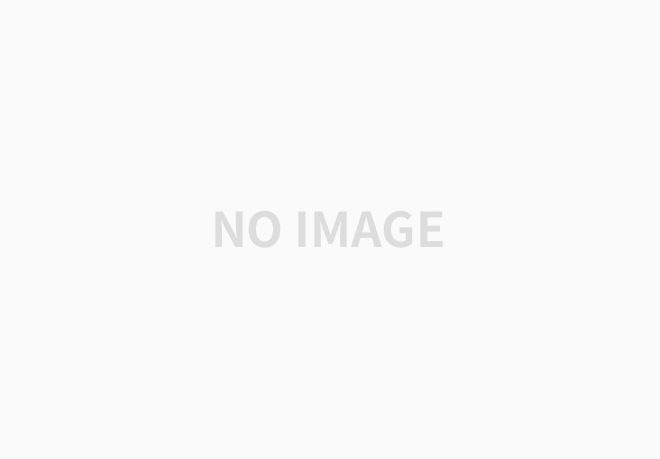
* Reference: Medina, Giovanna, et al. "Deep learning method for segmentation of rotator cuff muscles on MR images." Skeletal Radiology 50.4 (2021): 683-692.
728x90
728x90
댓글